The KCL researchers’ new models for predicting which patients will need hospitalization and breathing support may be useful for pathologists and clinical laboratory scientists
One more window into understanding the SARS-CoV-2 coronavirus may have just opened. A British study identified six distinct “clusters” of symptoms that the research scientists believe may help predict which patients diagnosed with COVID-19 will require hospitalization and respiratory support. If further research confirms these early findings, pathologists and medical laboratory managers may gain new tools to diagnose infections faster and more accurately.
Researchers from King’s College London (KCL) analyzed data gathered from the COVID Symptom Study App, a mobile-device application developed by health science company ZOE in collaboration with scientists and physicians at KCL and Massachusetts General Hospital, as well as:
- Harvard T.H. Chan School of Public Health,
- UTHealth,
- American Cancer Society,
- Dr. Susan Love Foundation,
- And others.
Launched in March in the United Kingdom and extended to the United States and Sweden, the app has attracted more than four million users who track their health and potential COVID symptoms on a daily basis.
Increased Accuracy in Predicting COVID-19 Hospitalizations
On July 17, 2020, the Centers for Disease Control and Prevention (CDC) published “Symptom Profiles of a Convenience Sample of Patients with COVID-19—United States, January–April 2020,” which identifies cough, fever, and shortness of breath as the most typical symptoms of COVID-19. However, the KCL study takes those findings a step further.
KCL researchers identified six distinct “types” of COVID-19, each distinguished by a particular cluster of symptoms. They include headaches, muscle pains, fatigue, diarrhea, confusion, loss of appetite, shortness of breath, and more. The researchers also found that COVID-19 disease progression and outcome also vary significantly between people, ranging from mild flu-like symptoms or a simple rash to severe or fatal conditions.
Using app data logged by 1,600 users in March and April, the researchers developed an algorithm that combined information on age, gender, body mass index (BMI), and pre-existing conditions with recorded symptoms from the onset of the illness through the first five days. The researchers then tested the algorithm using a second independent dataset of 1,000 users, logged in May.
In a news release, the KCL researchers identified the six clusters of symptoms as:
- Flu-like with No Fever: Headache, loss of smell, muscle pains, cough, sore throat, chest pain, no fever.
- Flu-like with Fever: Headache, loss of smell, cough, sore throat, hoarseness, fever, loss of appetite.
- Gastrointestinal: Headache, loss of smell, loss of appetite, diarrhea, sore throat, chest pain, no cough.
- Severe Level One, Fatigue: Headache, loss of smell, cough, fever, hoarseness, chest pain, fatigue.
- Severe Level Two, Confusion: Headache, loss of smell, loss of appetite, cough, fever, hoarseness, sore throat, chest pain, fatigue, confusion, muscle pain.
- Severe Level Three, Abdominal and Respiratory: Headache, loss of smell, loss of appetite, cough, fever, hoarseness, sore throat, chest pain, fatigue, confusion, muscle pain, shortness of breath, diarrhea, abdominal pain.
Using the data, the researchers were able to more accurately predict—78.8% versus 69.5%—which of the six symptom clusters placed patients at higher risk of requiring hospitalization and breathing support (ventilation or additional oxygen) than with prediction models based on personal characteristics alone. For example, nearly 50% of the patients in cluster six (Severe Level Three, Abdominal and Respiratory) ended up in the hospital, compared with 16% of those in cluster one (Flu-like with No Fever).
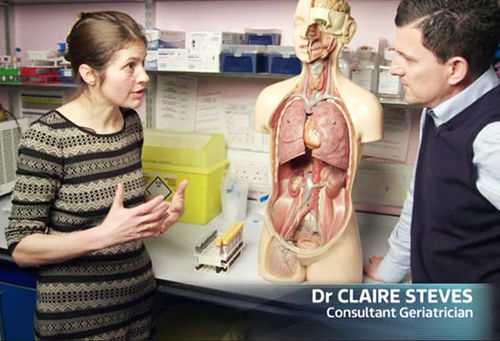
According to the Zoe website, the ongoing research is led by:
- Prof. Tim Spector, FMedSci, Professor of Genetic Epidemiology at King’s College London and Director of TwinsUK, an adult registry of twins in the United Kingdom;
- Andrew Chan, MD, Professor of Medicine at Harvard Medical School, Professor of Immunology and Infectious Diseases, Harvard T.H. Chan School of Public Health and Chief of the Clinical and Translational Epidemiology Unit, CTEU Massachusetts General Hospital; and
- Christopher Gardner, PhD, Rehnborg Farquhar Professor of Medicine at Stanford University Prevention Research Center.
The researchers published their study findings at medRxiv, titled, “Symptom Clusters in COVID-19: A Potential Clinical Prediction Tool from the COVID Symptom Study App.” The study has not yet undergone peer review.
Encouraging Everyone to Use the COVID-Symptom Study App
The study points out that—broadly speaking—people with cluster four, five, or six COVID-19 symptoms tended to be older and frailer and were more likely to be overweight and have pre-existing conditions, such as diabetes or lung disease, than those with cluster one, two, or three symptoms.
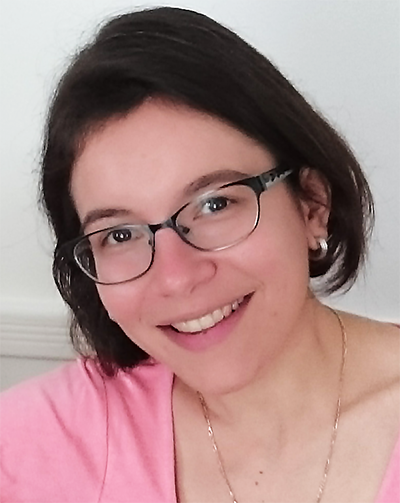
Tim Spector, FMedSci, Head of the Department of Twin Research and Genetic Epidemiology, and Professor of Genetic Epidemiology at King’s College London, encourages everyone to download the COVID Symptom Study app and help increase the data available to researchers.
“Data is our most powerful tool in the fight against COVID-19,” Spector said in the KCL news release. “We urge everyone to get in the habit of using the app daily to log their health over the coming months, helping us to stay ahead of any local hotspots or a second wave of infections.”
As the body of knowledge surrounding COVID-19 grows, clinical laboratory professionals would be well advised to remain informed on further research regarding not only the potential for COVID-19 variants to exist, but also the evolving guidance on infection prevention and testing.
—Andrea Downing Peck
Related Information:
Six Distinct ‘Types’ of COVID-19 Identified
Symptom Clusters in COVID19: A Potential Clinical Prediction Tool from the COVID Symptom Study App
Symptom Profile of a Convenience Sample of Patients with COVID-19–United States, January-April 2020
Trackbacks/Pingbacks