At hospitals where results of molecular COVID-19 testing can take up to several days to return, this new method for identifying potentially infected patients could improve triage
Frustrated by shortages of essential COVID-19 tests and supplies—as well by lengthy coronavirus test turn-around times—researchers at Weill Cornell Medicine have created an Artificial Intelligence (AI) algorithm that can use routine clinical laboratory test data to determine if a patient is infected with SARS-CoV-2, the coronavirus that causes the COVID-19 disease.
This is an important development because the turn-around-time (TAT) for common lab tests is generally much shorter than COVID-19 molecular diagnostics—such as real-time reverse transcription polymerase chain reaction (RT-PCR), currently the most popular coronavirus test—and certainly serological (antibody) diagnostics, which require an infection incubation time of as much as 10-14 days before testing.
Some RT-PCR diagnostic tests for COVID-19, which detect viral RNA on nasopharyngeal swab specimens, can take up to several days to return depending on the test and on the lab’s location. But routine medical laboratory tests generally return much sooner, often within minutes or hours, making this a potential game-changer for triaging infected patients.
Machine Learning Brings AI to COVID-19 Diagnostics
Advances in the use of AI in healthcare have led to the development of machine-learning algorithms that are being utilized as diagnostic tools for anatomic pathology, radiology, and for specific complex diseases, such as cancer. The Weill Cornell scientists wanted to see if alternative lab test results could be used by an algorithmic model to identify people infected with the SARS-CoV-2 coronavirus.
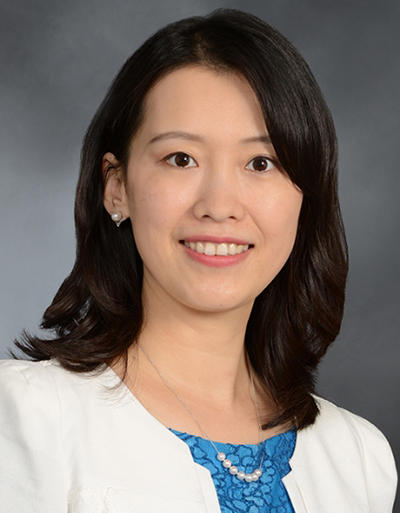
To perform the research, the team incorporated patients’ age, sex, and race, into a machine learning model that was based on results from 27 routine lab tests chosen from a total of 685 different tests ordered for the patients. The study included 3,356 patients who were tested for SARS-CoV-2 at New York-Presbyterian Hospital/Weill Cornell Medical Center between March 11 and April 29 of this year. The patients ranged in ages from 18 to 101 with the mean age being 56.4 years. Of those patients, 1,402 were RT-PCR positive and the remaining 1,954 were RT-PCR negative.
Using a machine-learning technique known as a gradient-boosting decision tree, the algorithm identified SARS-CoV-2 infections with 76% sensitivity and 81% specificity. When looking at only emergency department (ED) patients, the model performed even better with 80% sensitivity and 83% specificity. ED patients comprised just over half (54%) of the patients used for the study.
Weill Cornell Medicine Algorithm Could Lower False Negative Test Results
The algorithm also correctly identified patients who originally tested negative for COVID-19, but who tested positive for the coronavirus upon retesting within two days. According to the researchers, these results indicated their model could potentially decrease the amount of incorrect test results.
“We are thinking that those potentially false negative patients may demonstrate a different routine lab test profile that might be more similar to those that test positive,” Fei Wang, PhD, Assistant Professor of Healthcare Policy and Research at Weill Cornell Medicine and the study’s senior author, told Modern Healthcare. “So, it offers us a chance to capture those patients who are false negatives.”
The researchers validated their model by comparing the results with patients seen at New York Presbyterian Hospital/Lower Manhattan Hospital during the same time period. Among those patients, 496 were RT-PCR positive and 968 were negative and the algorithmic model performed with 74% specificity and 76% sensitivity.
In their study, published in the Oxford Academic journal Clinical Chemistry, titled, “Routine Laboratory Blood Tests Predict SARS-CoV-2 Infection Using Machine Learning,” the Weill Cornell Medicine scientists concluded that their research illustrated the algorithm could:
- preliminarily identify high-risk SARS-CoV-2 infected patients before RT-PCR results are available,
- risk stratify patients in the ED,
- select patients who need relatively urgent retesting if initial RT-PCR results are negative,
- help isolate infected patients earlier, and
- assist in the identification of SARS-CoV-2 infected patients in areas where RT-PCR testing is unavailable due to financial or supply constraints.
Early Results of Study Promising, But More Research is Needed
Wang noted that more research is needed on the algorithm and that he and his colleagues are currently working on ways to improve the model. They are hoping to test it with different conditions and geographies.
“Our model in the paper was built on data from when New York was at its COVID peak,” he told Modern Healthcare. “At that time, we were not doing wide PCR testing, and the patients who were getting tested were pretty sick.”
At the time of the study, the positivity rate for COVID-19 at New York-Presbyterian Hospital was in the 40% to 50% range. That was substantially higher than the current positivity rate, which is in the 2% to 3% range, Modern Healthcare reported.
“This model we built in a population in New York in a certain time period, so we can’t guarantee that it will work well universally,” Wang told Modern Healthcare.
It’s exciting to think that advances in software algorithms may one day make it possible to combine routine clinical laboratory testing and create diagnostics that identify diseases in ways the individual tests were not originally designed to do.
This study is an example that researchers in AI and informatics are working to bring new tools and diagnostic capabilities to clinical laboratories. Also, this is a demonstration of how a patient’s results from multiple other types of lab tests can by analyzed using AI and similar analytical algorithms to diagnose a health condition unrelated to the original reasons for performing those tests.
If this can be demonstrated with other diseases and health conditions, it would open up one more way that pathologists and clinical laboratory scientists can contribute to more accurate diagnoses and improved selection of the most appropriate therapies for individual patients.
—JP Schlingman
Related Information:
Routine Lab Tests Could Help Identify COVID-19 Patients
Routine Laboratory Blood Tests Predict SARS-CoV-2 Infection Using Machine Learning