McKinsey and Company Report Highlights Precision Medicine’s Advancements in Integrating Genetic Testing Results with Electronic Medical Records
New McKinsey report offers three market trends that could help clinical laboratories position themselves as front-runners in the race toward precision medicine With federal Medicare reporting and reimbursement programs now weighted heavily toward precision medicine practices that involve genetic testing to reveal predispositions to certain diseases, the trend is widely recognized as the future of U.S. healthcare. But are clinical laboratories and anatomic pathology groups prepared to take...Studies Show Potential for Google’s AI Tools to Improve Pathologist Accuracy and Efficiency
Computer-assisted analysis using Google’s LYNA algorithm shows significant gains in speed required to analyze stained lymph node slides and sensitivity of micrometastases detection in two recent studies
Anatomic pathologists understand the complexities of reviewing slides and samples for signs of cancer’s spread. Two studies involving a new artificial intelligence (AI) algorithm from Google (NASDAQ:GOOGL) claim their “deep learning” LYmph Node Assistant (LYNA) provides increases to both the speed at which pathologists can analyze slides and improved accuracy in detecting metastatic breast cancer within the slide samples used for the studies.
Google’s first study was published in the Archives of Pathology and Laboratory Medicine and investigated the accuracy of the algorithm using digital pathology slides. Google’s second study, published in The American Journal of Surgical Pathology, looked at how pathologists might harness the algorithm to improve workflows and use the tool in a clinical setting.
Medical laboratories and other diagnostics providers are already familiar with the improvement potential of automation and other technology-based approaches to diagnosis and analysis. Google’s LYNA is an example of how AI and machine learning improvements can serve as a supplement to—not a replacement for—the skills of experts at pathology groups and clinical laboratories.
Early research done by Google indicates that integrating LYNA into existing workflows could allow pathologists to spend less time analyzing slides for minute details. Instead, they could focus on other more challenging tasks while the AI analyzes gigapixels worth of slide data to highlight regions of concern in slides and samples for deeper manual inspection.
LYNA Achieves 99% Accuracy in Study of Metastatic Breast Cancer Detection
According to the research cited in a Google AI Blog post, roughly 25% of metastatic lymph node staging classifications would change if subjected to a second pathologic review. They further note that when faced with time constraints, detection sensitivity for small metastases on individual slides can be as low as 38%.
In findings published in Archives of Pathology and Laboratory Medicine, Google researchers analyzed whole slide images from hematoxylin-eosin-stained lymph nodes for 399 patients sourced from the Camelyon16 challenge dataset. Of those slides, researchers used 270 to train LYNA and the remaining 129 for analysis. They then compared the LYNA findings to those of an independent lab using a different scanner.
“LYNA achieved a slide-level area under the receiver operating characteristic (AUC) of 99% and a tumor-level sensitivity of 91% at one false positive per patient on the Camelyon16 evaluation dataset,” the researchers stated. “We also identified [two] ‘normal’ slides that contained micrometastases.”
Google’s algorithm later received an AUC of 99.6% on a secondary dataset.
“Artificial intelligence algorithms can exhaustively evaluate every tissue patch on a slide, achieving higher tumor-level sensitivity than, and comparable slide-level performance to, pathologists,” the researchers continued. “These techniques may improve the pathologist’s productivity and reduce the number of false negatives associated with morphologic detection of tumor cells.”
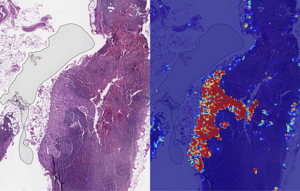
Left: sample view of a slide containing lymph nodes, with multiple artifacts: the dark zone on the left is an air bubble, the white streaks are cutting artifacts, the red hue across some regions are hemorrhagic (containing blood), the tissue is necrotic (decaying), and the processing quality was poor. Right: LYNA identifies the tumor region in the center (red), and correctly classifies the surrounding artifact-laden regions as non-tumor (blue). (Image and caption copyright: Google AI Blog.)
Faster Analysis through Software Assistance
Rapid diagnosis helps improve cancer outcomes. Yet, manually reviewing and analyzing complex digital slides is time-consuming. Time constraints might also lead to false negatives due to micrometastases or small suspicious regions that slip by pathologists undetected.
The Google research team of the study published in The American Journal of Surgical Pathology sought to gauge the impact LYNA might have on the histopathologic review of lymph nodes for trained pathologists. In their multi-reader multi-case study, researchers analyzed differences in both sensitivity of detecting micrometastases and the average review time per image using both computer-aided detection and unassisted detection for six pathologists across 70 slides.
Using the LYNA algorithm to identify and outline regions likely to contain tumors, the researchers found that sensitivity increased from 83% to 91%. The time to review slides also saw a significant reduction from 116 seconds in the unassisted mode to 61 seconds in the assisted mode—a time savings of roughly 47%.
“Although some pathologists in the unassisted mode were less sensitive than LYNA,” the researchers stated, “all pathologists performed better than the algorithm alone in regard to both sensitivity and specificity when reviewing images with assistance.”
The Future of Digital Pathology using LYNA
While the two studies show positive results, both studies also reveal shortcomings. Google highlighted both limited dataset sizes and simulated diagnostic workflows as potential concerns and areas on which to focus future studies.
Still, Google’s researchers believe that algorithms such as LYNA will help to power the future of diagnostics as healthcare in the digital era continues to mature. “We remain optimistic,” state the authors of the Google AI Blog post, “that carefully validated deep learning technologies and well-designed clinical tools can help improve both the accuracy and availability of pathologic diagnosis around the world.”
While other industries see risk in the growth of AI, both studies performed by researchers at Google show how computer-assisted workflows and machine learning could accentuate and bolster the skills of trained diagnosticians, such as anatomic pathologists and clinical laboratory technicians. By working to compensate for weak points in both human skill and computer reasoning, the outcome could be greater than either AI or humans can achieve separately.
—Jon Stone
Related Information:
Google Creates AI to Detect When Breast Cancer Spreads
Google Deep Learning Tool 99% Accurate at Breast Cancer Detection
Google’s AI Software Seeks to Detect Advanced Breast Cancer Better Than We Have Before
Google’s AI Is Better at Spotting Advanced Breast Cancer than Pathologists
Google AI Claims 99% Accuracy in Metastatic Breast Cancer Detection
Applying Deep Learning to Metastatic Breast Cancer Detection
Assisting Pathologists in Detecting Cancer with Deep Learning
Controversy Surrounding Memorial Sloan Kettering Cancer Center and Paige.AI Highlights Risks of Data Sharing and Monetization in Anatomic Pathology
Patient privacy, ethics of monetizing not-for-profit data, and questions surrounding industry conflicts appear after the public announcement of an arrangement to grant exclusive access to academic pathology slides and samples
Clinical laboratories and anatomic pathology groups already serve as gatekeepers for a range of medical data used in patient treatments. Glass slides, paraffin-embedded tissue specimens, pathology reports, and autopsy records hold immense value to researchers. The challenge has been how pathologists (and others) in a not-for-profit academic center could set themselves up to potentially profit from their exclusive access to this archived pathology material.
Now, a recent partnership between Memorial Sloan Kettering Cancer Center (MSK) and Paige.AI (a developer of artificial intelligence for pathology) shows how academic pathology laboratories might accomplish this goal and serve a similar gatekeeper role in research and development using the decades of cases in their archives.
The arrangement, however, is not without controversy.
New York Times, ProPublica Report
Following an investigative report from the New York Times (NYT) and ProPublica, pathologists and board members at MSK are under fire from doctors and scientists there who have concerns surrounding ethics, exclusivity, and profiting from data generated by physicians and but owned by MSK.
“Hospital pathologists have strongly objected to the Paige.AI deal, saying it is unfair that the founders received equity stakes in a company that relies on the pathologists’ expertise and work amassed over 60 years. They also questioned the use of patients’ data—even if it is anonymous—without their knowledge in a profit-driven venture,” the NYT article states.
Prominent members of MSK are facing scrutiny from the media and peers—with some relinquishing stakes in Paige.AI—as part of the backlash of the report. This is an example of the perils and PR concerns lab stakeholders might face concerning the safety of data sharing and profits made by medical laboratories and other diagnostics providers using patient data.
Controversy Surrounds Formation of Paige.AI/MSK Partnership
In February 2018, Paige.AI announced closing the deal on a $25-million round of Series A funding, and in gaining exclusive access to 25-million pathology slides and computational pathology intellectual property held by the Department of Pathology at Memorial Sloan Kettering. Coverage by TechCrunch noted that while MSK received an equity stake as part of the licensing agreement, they were not a cash investor.

TechCrunch lists David Klimstra, MD (left), Chairman of the Department of Pathology, MSK, and Thomas Fuchs, Dr.SC (right), Director of Computational Pathology in the Warren Alpert Center for Digital and Computational Pathology at MSK, as co-founders of Paige.AI. (Photo copyrights: New York Times/Thomas Fuchs Lab.)
Creation of the company involved three hospital insiders and three additional board members with the hospital itself established as part owner, according to STAT.
Unnamed officials told the NYT that board members at MSK only invested in Paige.AI after earlier efforts to generate outside interest and investors were unsuccessful. NYT’s coverage also notes experts in non-profit law and corporate governance have raised questions as to compliance with federal and state laws that govern nonprofits in light of the Paige.AI deal.
Growing Privacy Fallout and Potential Pitfalls for Medical Labs
The original September 2018 NYT coverage noted that Klimstra intends to divest his ownership stake in Paige.AI. Later coverage by NYT in October, notes that Democrat Representative Debbie Dingell of Michigan submitted a letter questioning details about patient privacy related to Paige.AI’s access to MSK’s academic pathology resources.
Privacy continues to be a focus for both media and regulatory scrutiny as patient data continues to fill electronic health record (EHR) systems as well as research and commercial databases. Dark Daily recently covered how University of Melbourne researchers demonstrated how easily malicious parties might reidentify deidentified data. (See “Researchers Easily Reidentify Deidentified Patient Records with 95% Accuracy; Privacy Protection of Patient Test Records a Concern for Clinical Laboratories”, October 10, 2018.)
According to the NYT, MSK also issued a memo to employees announcing new restrictions on interactions with for-profit companies with a moratorium on board members investing in or holding board positions in startups created within MSK. The nonprofit further noted it is considering barring hospital executives from receiving compensation for their work on outside boards.
However, MSK told the NYT this only applies to new deals and will not affect the exclusive deal between Paige.AI and MSK.
“We have determined,” MSK wrote, “that when profits emerge through the monetization of our research, financial payments to MSK-designated board members should be used for the benefit of the institution.”
There are no current official legal filings regarding actions against the partnership. Despite this, the arrangement—and the subsequent fallout after the public announcement of the arrangement—serve as an example of pitfalls medical laboratories and other medical service centers considering similar arrangements might face in terms of public relations and employee scrutiny.
Risk versus Reward of Monetizing Pathology Data
While the Paige.AI situation is only one of multiple concerns now facing healthcare teams and board members at MSK, the events are an example of risks pathologists take when playing a role in a commercial enterprise outside their own operations or departments.
In doing so, the pathologists investing in and shaping the deal with Paige.AI brought criticism from reputable sources and negative exposure in major media outlets for their enterprise, themselves, and MSK as a whole. The lesson from this episode is that pathologists should tread carefully when entertaining offers to access the patient materials and data archived by their respective anatomic pathology and clinical laboratory organizations.
—Jon Stone
Related Information:
Sloan Kettering’s Cozy Deal with Start-Up Ignites a New Uproar
Paige.AI Nabs $25M, Inks IP Deal with Sloan Kettering to Bring Machine Learning to Cancer Pathology
Sloan Kettering Executive Turns Over Windfall Stake in Biotech Start-Up
Cancer Center’s Board Chairman Faults Top Doctor over ‘Crossed Lines’
Memorial Sloan Kettering, You’ve Betrayed My Trust
LVHN Patient Data Not Shared with For-Profit Company in Sloan Kettering Trials
IBM’s Watson Not Living Up to Hype, Wall Street Journal and Other Media Report; ‘Dr. Watson’ Has Yet to Show It Can Improve Patient Outcomes or Accurately Diagnose Cancer
Wall Street Journal reports IBM losing Watson-for-Oncology partners and clients, but scientists remain confident artificial intelligence will revolutionize diagnosis and treatment of disease
What happens when a healthcare revolution is overhyped? Results fall short of expectations. That’s the diagnosis from the Wall Street Journal (WSJ) and other media outlets five years after IBM marketed its Watson supercomputer as having the potential to “revolutionize” cancer diagnosis and treatment.
The idea that artificial intelligence (AI) could be used to diagnose cancer and identify appropriate therapies certainly carried with it implications for clinical laboratories and anatomic pathologists, which Dark Daily reported as far back as 2012. It also promised to spark rapid growth in precision medicine. For now, though, that momentum may be stalled.
“Watson can read all of the healthcare texts in the world in seconds,” John E. Kelly III, PhD, IBM Senior Vice President, Cognitive Solutions and IBM Research, told Wired in 2011. “And that’s our first priority, creating a ‘Dr. Watson,’ if you will.”
However, despite the marketing pitch, the WSJ investigation published in August claims IBM has fallen far short of that goal during the past seven years. The article states, “More than a dozen IBM partners and clients have halted or shrunk Watson’s oncology-related projects. Watson cancer applications have had limited impact on patients, according to dozens of interviews with medical centers, companies and doctors who have used it, as well as documents reviewed by the Wall Street Journal.”
Anatomic pathologists—who use tumor biopsies to diagnose cancer—have regularly wondered if IBM’s Watson would actually help physicians do a better job in the diagnosis, treatment, and monitoring of cancer patients. The findings of the Wall Street Journal show that Watson has yet to make much of a positive impact when used in support of cancer care.
The WSJ claims Watson often “didn’t add much value” or “wasn’t accurate.” This lackluster assessment is blamed on Watson’s inability to keep pace with fast-evolving treatment guidelines, as well as its inability to accurately evaluate reoccurring or rare cancers. Despite the more than $15 billion IBM has spent on Watson, the WSJ reports there is no published research showing Watson improving patient outcomes.
Lukas Wartman, MD, Assistant Professor, McDonnell Genome Institute at the Washington University School of Medicine in St. Louis, told the WSJ he rarely uses the Watson system, despite having complimentary access. IBM typically charges $200 to $1,000 per patient, plus consulting fees in some cases, for Watson-for-Oncology, the WSJ reported.
“The discomfort that I have—and that others have had with using it—has been the sense that you never know how much faith you can put in those results,” Wartman said.
Rudimentary Not Revolutionary Intelligence, STAT Notes
IBM’s Watson made headlines in 2011 when it won a head-to-head competition against two champions on the game show “Jeopardy.” Soon after, IBM announced it would make Watson available for medical applications, giving rise to the idea of “Dr. Watson.”
In a 2017 investigation, however, published on STAT, Watson is described as in its “toddler stage,” falling far short of IBM’s depiction of Watson as a “digital prodigy.”
“Perhaps the most stunning overreach is in [IBM’s] claim that Watson-for-Oncology, through artificial intelligence, can sift through reams of data to generate new insights and identify, as an IBM sales rep put it, ‘even new approaches’ to cancer care,” the STAT article notes. “STAT found that the system doesn’t create new knowledge and is artificially intelligent only in the most rudimentary sense of the term.”
STAT reported it had taken six years for data engineers and doctors to train Watson in just seven types of cancers and keep the system updated with the latest knowledge.
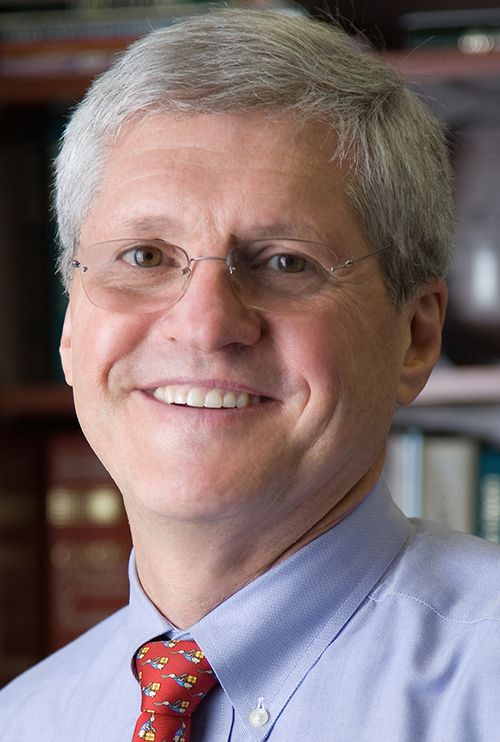
“It’s been a struggle to update, I’ll be honest,” Mark Kris, MD, oncologist at Memorial Sloan Kettering Cancer Center in New York and lead Watson trainer, told STAT. “Changing the system of cognitive computing doesn’t turn on a dime like that. You have to put in the literature, you have to put in the cases.” (Photo copyright: Physician Education Resource.)
Watson Recommended Unsafe and Incorrect Treatments, STAT Reported
In July 2018, STAT reported that internal documents from IBM revealed Watson had recommended “unsafe and incorrect” cancer treatments.
David Howard, PhD, Professor, Health Policy and Management, Rollins School of Public Health at Emory University, blames Watson’s failure in part to the dearth of high-quality published research available for the supercomputer to analyze.
“IBM spun a story about how Watson could improve cancer treatment that was superficially plausible—there are thousands of research papers published every year and no doctor can read them all,” Howard told HealthNewsReview.org. “However, the problem is not that there is too much information, but rather there is too little. Only a handful of published articles are high-quality, randomized trials. In many cases, oncologists have to choose between drugs that have never been directly compared in a randomized trial.”
Howard argues the news media needs to do a better job vetting stories touting healthcare breakthroughs.
“Reporters are often susceptible to PR hype about the potential of new technology—from Watson to ‘wearables’—to improve outcomes,” Howard said. “A lot of stories would turn out differently if they asked a simple question: ‘Where is the evidence?’”
Peter Greulich, a retired IBM manager who has written extensively on IBM’s corporate challenges, told STAT that IBM would need to invest more money and people in the Watson project to make it successful—an unlikely possibility in a time of shrinking revenues at the corporate giant.
“IBM ought to quit trying to cure cancer,” he said. “They turned the marketing engine loose without controlling how to build and construct a product.”
AI Could Still Revolutionize Precision Medicine
Despite the recent negative headlines about Watson, AI continues to offer the promise of one day changing how pathologists and physicians work together to diagnose and treat disease. Isaac Kohane, MD, PhD, Chairman of the Biomedical Informatics Program at Harvard Medical School, told Bloomberg that IBM may have oversold Watson, but he predicts AI one day will “revolutionize medicine.”
“It’s anybody’s guess who is going to be the first to the market leader in this space,” he said. “Artificial intelligence and big data are coming to doctors’ offices and hospitals. But it won’t necessarily look like the ads on TV.”
How AI and precision medicine plays out for clinical laboratories and anatomic pathologists is uncertain. Clearly, though, healthcare is on a path toward increased involvement of computerized decision-making applications in the diagnostic process. Regardless of early setbacks, that trend is unlikely to slow. Laboratory managers and pathology stakeholders would be wise to keep apprised of these developments.
—Andrea Downing Peck
Related Information:
IBM Pitched its Watson Supercomputer as a Revolution in Cancer Care. It’s Nowhere Close
IBM’s Watson Wins Jeopardy! Next Up: Fixing Health Care
IBM’s Watson Supercomputer Wins Practice Jeopardy Round
MD Anderson Cancer Center’s IBM Watson Project Fails, and So Did the Journalism Related to It
What Went Wrong with IBM’s Watson?
IBM’s Watson Failed Against Cancer but AI Still Has Promise
Pathologists Take Note: IBM’s Watson to Attack Cancer with Help of WellPoint and Cedars-Sinai