Machine Learning System Catches Two-Thirds More Prescription Medication Errors than Existing Clinical Decision Support Systems at Two Major Hospitals
Researchers find a savings of more than one million dollars and prevention of hundreds, if not thousands, of adverse drug events could have been had with machine learning system
Support for artificial intelligence (AI) and machine learning (ML) in healthcare has been mixed among anatomic pathologists and clinical laboratory leaders. Nevertheless, there’s increasing evidence that diagnostic systems based on AI and ML can be as accurate or more accurate at detecting disease than systems without them.
Dark Daily has covered the development of artificial intelligence and machine learning systems and their ability to accurately detect disease in many e-briefings over the years. Now, a recent study conducted at Brigham and Women’s Hospital (BWH) and Massachusetts General Hospital (MGH) suggests machine learning can be more accurate than existing clinical decision support (CDS) systems at detecting prescription medication errors as well.
The researchers published their findings in the Joint Commission Journal on Quality and Patient Safety, titled, “Using a Machine Learning System to Identify and Prevent Medication Prescribing Errors: A Clinical and Cost Analysis Evaluation.”
A Retrospective Study
The study was partially retrospective in that the researchers compiled past alerts generated by the CDS systems at BWH and MGH between 2009-2011 and added them to alerts generated during the active part of the study, which took place from January 1, 2012 to December 31, 2013, for a total of five years’ worth of CDS alerts.
They then sent the same patient-encounter data that generated those CDS alerts to a machine learning platform called MedAware, an AI-enabled software system developed in Ra’anana, Israel.
MedAware was created for the “identification and prevention of prescription errors and adverse drug effects,” notes the study, which goes on to state, “This system identifies medication issues based on machine learning using a set of algorithms with different complexity levels, ranging from statistical analysis to deep learning with neural networks. Different algorithms are used for different types of medication errors. The data elements used by the algorithms include demographics, encounters, lab test results, vital signs, medications, diagnosis, and procedures.”
The researchers then compared the alerts produced by MedAware to the existing CDS alerts from that 5-year period. The results were astonishing.
According to the study:
- “68.2% of the alerts generated were unique to the MedAware system and not generated by the institutions’ CDS alerting system.
- “Clinical outlier alerts were the type least likely to be generated by the institutions’ CDS—99.2% of these alerts were unique to the MedAware system.
- “The largest overlap was with dosage alerts, with only 10.6% unique to the MedAware system.
- “68% of the time-dependent alerts were unique to the MedAware system.”
Perhaps even more important was the results of the cost analysis, which found:
- “The average cost of an adverse event potentially prevented by an alert was $60.67 (range: $5.95–$115.40).
- “The average adverse event cost per type of alert varied from $14.58 (range: $2.99–$26.18) for dosage outliers to $19.14 (range: $1.86–$36.41) for clinical outliers and $66.47 (range: $6.47–$126.47) for time-dependent alerts.”
The researchers concluded that, “Potential savings of $60.67 per alert was mainly derived from the prevention of ADEs [adverse drug events]. The prevention of ADEs could result in savings of $60.63 per alert, representing 99.93% of the total potential savings. Potential savings related to averted calls between pharmacists and clinicians could save an average of $0.047 per alert, representing 0.08% of the total potential savings.
“Extrapolating the results of the analysis to the 747,985 BWH and MGH patients who had at least one outpatient encounter during the two-year study period from 2012 to 2013, the alerts that would have been fired over five years of their clinical care by the machine learning medication errors identification system could have resulted in potential savings of $1,294,457.”
Savings of more than one million dollars plus the prevention of potential patient harm or deaths caused by thousands of adverse drug events is a strong argument for machine learning platforms in diagnostics and prescription drug monitoring.
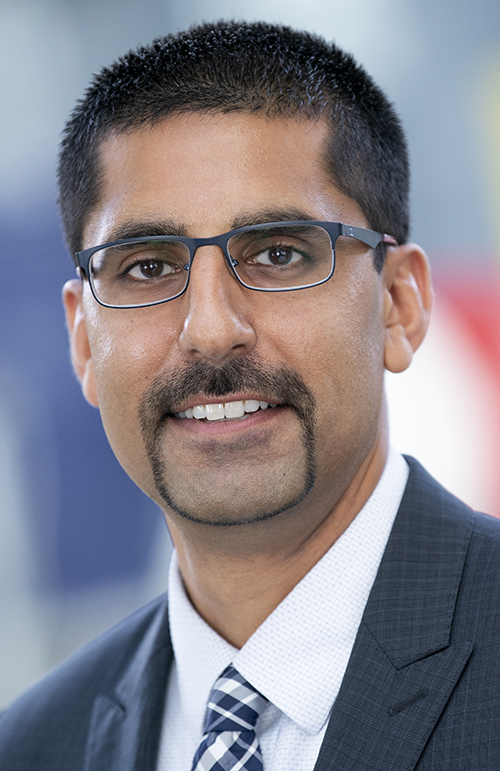
Researchers Say Current Clinical Decision Support Systems are Limited
Machine learning is not the same as artificial intelligence. ML is a “discipline of AI” which aims for “enhancing accuracy,” while AI’s objective is “increasing probability of success,” explained Tech Differences.
Healthcare needs the help. Prescription medication errors cause patient harm or deaths that cost more than $20 billion annually, states a Joint Commission news release.
CDS alerting systems are widely used to improve patient safety and quality of care. However, the BWH-MGH researchers say the current CDS systems “have a variety of limitations.” According to the study:
- “One limitation is that current CDS systems are rule-based and can thus identify only the medication errors that have been previously identified and programmed into their alerting logic.
- “Further, most have high alerting rates with many false positives, resulting in alert fatigue.”
Alert fatigue leads to physician burnout, which is a big problem in large healthcare systems using multiple health information technology (HIT) systems that generate large amounts of alerts, such as: electronic health record (EHR) systems, hospital information systems (HIS), laboratory information systems (LIS), and others.
Commenting on the value of adding machine learning medication alerts software to existing CDS hospital systems, the BWH-MGH researchers wrote, “This kind of approach can complement traditional rule-based decision support, because it is likely to find additional errors that would not be identified by usual rule-based approaches.”
However, they concluded, “The true value of such alerts is highly contingent on whether and how clinicians respond to such alerts and their potential to prevent actual patient harm.”
Future research based on real-time data is needed before machine learning systems will be ready for use in clinical settings, HealthITAnalytics noted.
However, medical laboratory leaders and pathologists will want to keep an eye on developments in machine learning and artificial intelligence that help physicians reduce medication errors and adverse drug events. Implementation of AI-ML systems in healthcare will certainly affect clinical laboratory workflows.
—Donna Marie Pocius
Related Information:
AI and Healthcare: A Giant Opportunity
Machine Learning System Accurately Identifies Medication Errors
Differences Between Machine Learning and Artificial Intelligence