University of Pittsburgh Pathologists Create World Tumor Registry to Assist Medical Professionals in the Identification and Diagnosis of Cancers
As the cancer registry expands it will increasing become more useful to anatomic pathologists, histopathologists, oncologists, and even clinical laboratories
Oncologists, histopathologists, anatomic pathologists, and other cancer physicians now have a powerful new Wikipedia-style tumor registry to help them with their diagnoses and in educating patients on their specific types of cancer. Clinical laboratory managers may find it useful to understand the value this searchable database, and it can help their staff pathologists as well.
Free to use by both physicians and patients the World Tumor Registry (WTR) is designed “to minimize diagnostic errors by giving doctors a searchable online database of cancers that have been collected and categorized with cellular images collected from around the world,” Pittsburg-Post Gazette reported.
Prompt, accurate cancer diagnoses offer cancer patients the best chance for optimal treatment outcomes. However, many medical professionals around the globe do not have the training and resources to offer superior cancer diagnoses. That deficiency can translate to inferior treatment options and lower survival rates among cancer patients.
To help improve cancer diagnoses, pathologist Yuri E. Nikiforov, MD, PhD, Division Director, Molecular and Genomic Pathology, Vice Chair of the Department of Pathology, and Professor of Pathology, University of Pittsburgh, developed the WTR to provide educational and practical resources for individuals and organizations involved in cancer research.
Officially announced at the United States and Canadian Academy of Pathology (USCAP) annual convention, the WTR is an open-access catalog of digital microscopic images of human cancer types and subtypes.
The lower cost of technology and improved speed of access via the internet are technologies enabling this effort.
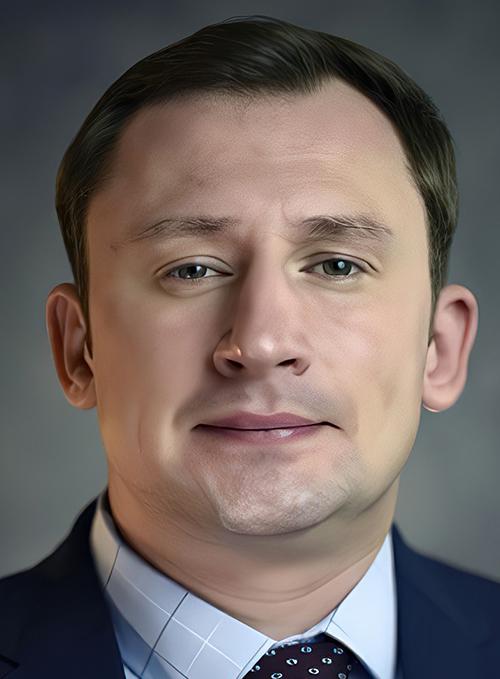
“We are creating sort of a Wikipedia for cancer images,” said Alyaksandr V. Nikitski, MD, PhD (above), Research Assistant Professor of Pathology, Division of Molecular and Genomic Pathology at Pittsburg School of Medicine and Administrative Director of the WTR, in an exclusive interview with Dark Daily. “Anyone in the world, if they can access the internet, can look at the well-annotated, diagnostic digital slides of cancer,” said Nikitski. Clinical laboratories may also find this new pathology tool useful. (Photo copyright: Alyaksandr V. Nikitski)
Minimizing Diagnostic Errors
Based in Pittsburgh, the WTR is freely available to anyone for viewing digital pathology slides of known cancer tumors as well as borderline and questionable cases. On the website, individuals can search for pictures of tumors in the registry by diagnosis, specific cohorts, and by microscopic features. Individuals may search further by tumor type and subtype to receive a picture of related tumors.
According to the WTR website, the mission of the nonprofit “is to minimize diagnostic errors, eliminate inequality in cancer recognition, diagnosis, and treatment in diverse populations, and improve outcomes by increasing access to the diagnostic pathology expertise and knowledge of microscopic characteristics of cancers that occur in different geographic, environmental, and socio-economic settings.”
This new comprehensive initiative will eventually encompass cancer images from all over the world.
“Let’s assume that I am a pathologist or a trainee who has little experience, or I don’t have access to collections of atypical tumors,” Nikitski explained. “I can view tumor collections online [in the WTR database] and check how typical and rare tumors look in various geographic regions and environmental settings.”
Once an image of a slide is selected, users will then receive a brief case history of the tumor in addition to such data as the age of the patient, their geographic location, sex, family history of the disease, and the size and stage of the tumor.
Increasing Probability of Correct Diagnosis
Pathologists and clinicians may also predict the probability of a particular diagnosis by searching under the microscopic feature of the database. This feature utilizes an innovative classifier known as PathDxFinder, where users may compare a slide from their lab to slides in the database by certain criteria. This includes:
- Patient age
- Family history of the cancer
- Number of nodules
- Tumor capsule/interface with normal tissue
- Growth pattern
- Cell shape
- Cytoplasm
- Overall nuclear appearance
- Nuclear size, shape, contours, pseudoinclusions and chromatin
- Nucleoli
- Tumor necrosis and/or increased mitosis
- Psammoma bodies
- Stroma
- Any unusual tumor features
After completing the questions above, the user presses the “predict diagnosis” button to receive the probability of cancer and most likely diagnosis based on the answers provided in the questionnaire.
WTR Editorial Boards
The WTR represents collections for each type of cancer site, such as lung or breast. A chairperson and editorial board are responsible for reviewing submitted slides before they are placed online. The editorial boards include 20 pathologists who are experts in diagnosing cancer categories, Nikitski explained.
Thousands of identified microscopic whole slide images (WSI) representing various types of cancer are deposited by the editors and other contributors to the project. The editorial board then carefully analyzes and compiles the data before posting the images for public viewing.
The editorial boards are located in five world regions:
- Africa and the Middle East
- Asia and Oceania
- Central and South America
- North America and Europe
- Northern Asia
Any physicians or pathologists can contribute images to the database, by “simply selecting the editor of their region on the website, writing their name, and asking if they can submit tumor cases,” Nikitski stated.
“We have established a platform that allows pathologists to contact editors who are in the same geographic region,” he added.
Helping Physicians Identify Cancer Types
In a YouTube video, Nikiforov states that the WTR is an “educational nonprofit organization rooted in [the] beliefs that every cancer patient deserves accurate and timely diagnosis as the first and essential step in better treatment and outcomes.”
“We believe this can be achieved only when modern diagnostic tools and technologies are freely available to every physician and pathologist. Only when we understand how microscopic features of cancer vary in different geographic, environmental and ethnic populations, and only by integrating histopathology with clinical immunohistochemical and molecular genetic information for every cancer type,” he stated.
Since patient privacy is important, the database contains only basic data about patients, and all patient information is protected.
Launched in March, there are currently more than 400 thyroid tumor slides available to view in the online database. At the time of the announcement, the WTR platform was planned to be implemented in three phases:
- Thyroid cancer (released in March of this year).
- Lung cancer and breast cancer (anticipated to be completed by the third quarter of 2026).
- Remaining cancers, including brain, soft tissue and bone, colorectal, head and neck, hematolymphoid, female genital, liver, pancreatic, prostate and male genital, skin, urinary system, pediatric, other endocrine cancers, and rare cancers (anticipated to be completed by the end of 2029).
“We believe that this resource will help physicians and pathologists practicing in small or big or remote medical centers to learn how cancer looks under a microscope in their own communities,” Nikiforov said in the video. “We also see WTR as a platform that connects physicians and scientists from different parts of the world who can work together to better understand and treat cancer.”
Catalogs like the World Tumor Registry might potentially create a pool of information that that could be mined by analytical and artificial intelligence (AI) platforms to ferret out new ways to improve the diagnosis of certain types of cancer and even enable earlier diagnoses.
“It is an extremely useful resource,” Nikitski said.
Anatomic pathologists will certainly find it so. And clinical laboratory managers may find the information useful as well when interacting with histopathologists and oncologists.
—JP Schlingman
Related Information:
“Free for the World:” Pittsburgh Pathologist Prepares to Launch a Wikipedia for Cancer
Video: Message from the Founder and President of the World Tumor Registry